Craft Data Science Insights: A 40-Minute Exploration – Session #4
Date/Time: March 28, 2024 | 6:00–7:00 pm
Location: Hybrid Event - Virtual/Beering Hall 1222
Get ready to be inspired! This miniseries brings together five brilliant young minds from education, psychology, learning science, and survey methodology. They’ll share their innovative solutions to challenges, powered by AI and data science.
How Early, Accurate, and Fair Can We Predict Student Learning in Foundational STEM Courses?
Dr. Ying Cheng, University of Notre Dame
Machine learning algorithms have been widely used for educational applications, such as predicting who might be at risk of failing a course, or sometimes referred to as identifying at-risk students. Existing research has focused on developing algorithms to improve the timeliness and accuracy of such predictions. Such a focus implies limited learning activity data and an overreliance on demographic background variables, which brings forth fairness concerns. In this talk, I will first introduce two studies in which we examined the utility of predictive modeling in early identification of at-risk students in two foundational STEM courses, respectively: organic chemistry (college level) and statistics (high school level). Then I will show how we evaluated the performance of the predictive models using various fairness metrics and explored the efficacy of different bias mitigation strategies in the second study. Finally, I will discuss the implications of the findings in terms of fair and explainable AI.
This hybrid event will be offered virtually and in Beering Hall 1222.
View event recording: https://youtu.be/T1xH3S_PAFo
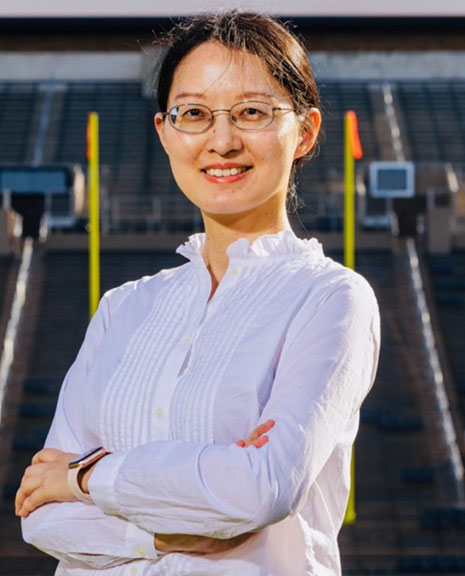
About the Presenter
Dr. Ying (“Alison”) Cheng is Professor of Quantitative Psychology and Fellow of the Institute for Educational Initiatives at University of Notre Dame. She is also the Associate Director of Education at the Lucy Family Institute of Data and Society at Notre Dame. She received her M.S.in Statistics and Ph.D. in Quantitative Psychology from the University of Illinois at Urbana-Champaign. Her research focuses on two areas: 1) Psychological and educational measurement; 2) Learning analytics. In the first area, she is interested in theoretical development and applications of item response theory (IRT), including computerized adaptive testing (CAT), test fairness, classification accuracy and consistency with licensure/certification exams, and cognitive diagnostic models and their applications in classroom assessments. In the second area, she is interested in applying data mining techniques to large-scale, multi-modal data, and building fair and accurate machine learning models for education applications. She has published over 70 peer-reviewed journal articles and her work has received funding support from national agencies such as NSF and IES.
She served as the Editor of the British Journal of Mathematical and Statistical Psychology for five years (2019 – 2023), and the Associate Editor of the American Educational Research Journal (2015 – 2018). In 2009 she received the Bradley Hanson Award for Contributions to Educational Measurement, and in 2012 the Jason Millman Promising Measurement Scholar Award from the National Council of Measurement in Education. In 2014 she received the faculty CAREER award from the National Science Foundation. She is an elected fellow of the Society of Multivariate and Experimental Psychology (2017), Association for Psychological Science (2019), and American Psychological Association (2023).
Dr. Ying Cheng
Professor
Department of Psychology
Fellow of the Institute for Educational Initiatives
Associate Director of Education at the Lucy Family Institute of Data and Society
University of Notre Dame
Contact: Hua-Hua Chang, chang606@purdue.edu